![]() A lot of what an analyst does is perform linear analysis. These analyses are guaranteed to produce human-readable stories, even if they aren't insightful. The world in which we live is not linear. In the book, 17 Equations That Changed The World, the author, Ian Stewart, selects only three equations that are linear, the rest are non-linear. This shows the limitations of linear analysis in explaining the world around us. A lot of what we experience in life is non-linear, from the flight of a ball in the air (parabolic) to the growth of your savings (exponential). |
||
What's true of the physical world is also true of the human brain too. One example is the way in which our brains use non-linear relationships to evaluate choices. One of the foundational tenets in the field of Behavioral Economics is Kahneman and Tversky's Prospect Theory and Loss Aversion.
Loss aversion describes the non-linear relationship between the value associated with gain of an item versus the value associated with loss of the same item. We would rather not lose something than find that same thing. Whether we are conscious of this or not, our brains use it every day when we evaluate choices, protecting what we own is a greater driver of behavior than gaining new things, it is one reason why the insurance market is so large. |
||
A good analyst will over lay this non-linear understanding of the world when interpreting findings, however it would be useful if analytics software could allow for human-readable non-linear analytics (it's what makes Support Vector Machines so powerful, yet so indecipherable). |
The Power of Data (Engineering)
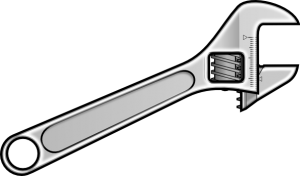
The secret to successful analytics lies in data engineering, as much as algorithm selection. Sure, there are exceptions to this. No doubt there are times when only one specific algorithm will work for a particular set of data. However, we believe there is no substitute for sound data engineering.
Data engineering is the process of feature creation. Features in the data are what an analytics algorithm will use to making predictions or estimation. Depending on how features are being created by a data engineering process will ultimately determine how human-readable the final models will be. It is easy to go from data engineering to data over-engineering.
An example of the pitfalls of data over-engineering is in the use of Support Vector Machines. The SVM classification algorithm is very powerful, it achieves this by a) only focusing on the handful of data points which defy a simple black-and-white separation of the data and b) performing data engineering that exposes powerful data features but which might not make sense to the ordinary person. For some use cases this is acceptable, but SVM classifications could easily enter the territory of "snake oil". SVM are an expert-user tool and the end user has to trust the person performing the analytics, because the outputs become too complex to explain in simple human terms.
Human readable models are a current focus of KL. We are in the middle of building out our data engineering functionality to allow users to create human-readable features from many different data-structure types. These new features will improve the power of KL's analytics algorithms without rendering them exclusively machine-readable.